“A perfect model does not exist, and never will,” said Jos Gheerardyn in an interview with Bloovi. A year later, his sentiments remain the same, if not even stronger, as AI and ML continue to penetrate deeper into the realm of finance and banking. He explains that an algorithm or model that is mathematically correct can still yield wrong results if the context changes. Suppose that you predict the weather today with an algorithm that was trained on meteorological data from the 19th century – you will see that the model does not perform very well, because the climate has changed, and the data you used for training does not accurately represent the current weather dynamics. The same applies to banking firms whose reliance on models for predictive decision-making increases constantly. Firms have to continuously monitor algorithms to ensure that they remain fit for purpose.
Jos Gheerardyn founded Yields with Sebastien Viguie in 2017. Since then he has dedicated his life’s work to developing a comprehensive solution that allows model developers and validators to stay in control of their algorithms. Having worked in model risk management, Jos’ first-hand experience helped define his vision and mission as a CEO.
Bridging the market gap
“I found that there were a lot of tools to build models and algorithms, while the challenge of keeping analytics reliably in production remained unaddressed.” Jos and Sebastien’s realization, and their profound understanding of the pain and struggles of carrying out manual validation and monitoring, motivated him to lead the development of what is now a highly-acclaimed model risk management platform, designed to help banks manage model risk effectively and efficiently. It does this thanks to automation, which significantly shortens the amount of time banks need to spend on validating models and generating model documentation.
Responsible AI and Yields
The AI industry is booming, and companies across different sectors are harnessing the potential of advanced analytics. AI’s time may have finally come, but algorithms are not created equal. AI still faces practical challenges such as bias and explainability, which require significant human effort and supervision. “In the financial services sector, regulatory bodies have issued standards for the management of model risk. But today, model risk has become a universal challenge across other domains that employ AI and machine learning.” Jos explains that more attention should be given to ensuring the robustness and accuracy of these models.
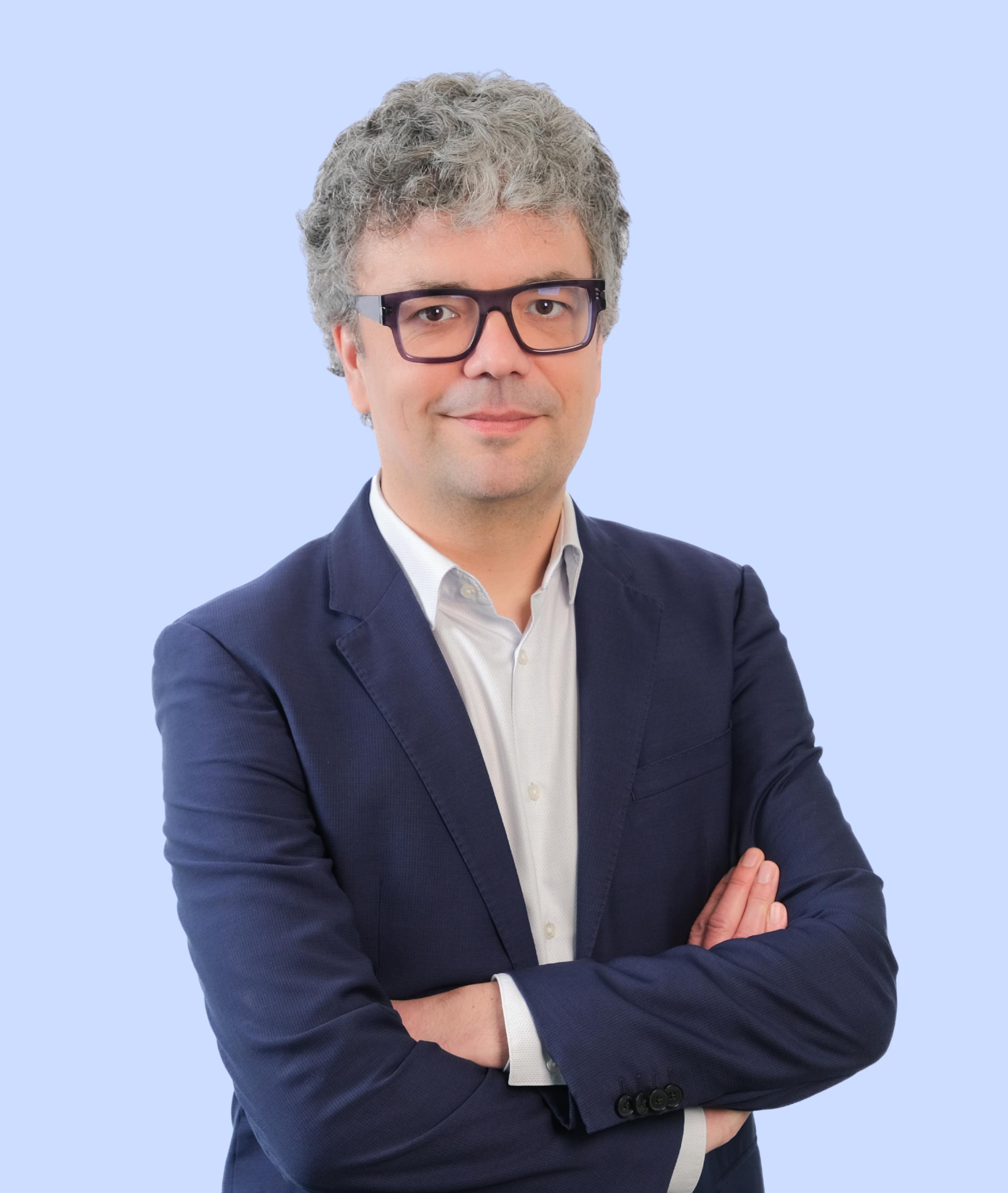
Companies operating in high-risk environments, including healthcare, human resources, and cybersecurity, are increasingly recognizing the paramount importance of conducting rigorous model testing to ensure the optimal performance and reliability of their models. Our vision has always been to create more awareness around the potential risks algorithms pose, and help companies to manage these risks and ensure that AI applications are safe for all. That is what’s driving our team to continuously innovate and improve our technology solutions.