In April 2021, the EU published a draft proposal on Trustworthy AI. As a company that envisions embedding trust in the algorithms of our clients, this proposed regulation is highly imperative to us at Yields. On that note, we had an interview with our CEO, Jos Gheerardyn, to elicit his thoughts on the draft proposal.
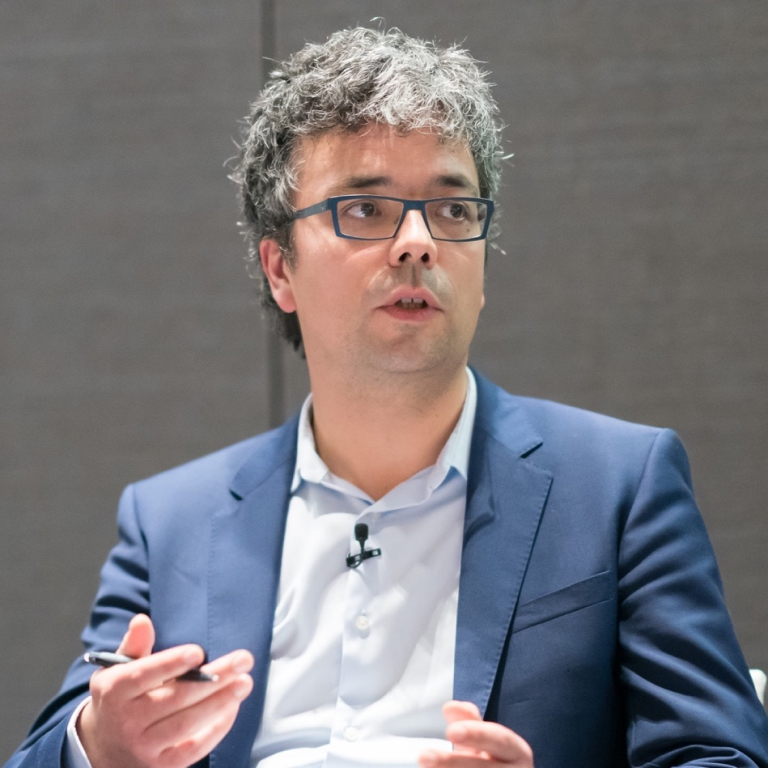
Jos Gheerardyn, CEO at Yields
Chidinma: Why is regulation from the EU on trustworthy AI needed?
Jos:
The proposed regulation from the European Commission to ensure the development of human-centric, inclusive and trustworthy AI is very much needed, not only in Europe but also in other continents. Its main purpose is to protect people, their human rights, and societal values while harnessing the potential of AI. If a company builds an AI application, and for some reason the application ends up having adverse consequences on both the lives of the builder and the users, then the AI application does not work properly.
For example, a recent study exposed Racial Bias in Pulse Oximetry Measurement. Researchers discovered that the case-study sensors used in measuring oxygen and blood levels do not work well with people of colour. In this specific case, the sensors were badly trained or calibrated to measure oxygen levels. Because of this, many people of colour did not receive sufficient medical treatment, owing to the fact that their oxygen levels were mistaken to be ‘high enough’. Especially in this era of COVID-19, this kind of misdiagnosis could result in much more casualties all because of a wrongly trained algorithm.
This is obviously the kind of risk that the EU is trying to mitigate by regulating specific use cases of AI. Quite similar to what happened with consumer goods; they have to be certified, with a certain level of safety guaranteed before they become available to the public for consumption.
Chidinma: What are according to you the main requirements the EU imposes to foster trustworthy AI?
Jos:
One of the main insights that stand out in the EU’s draft proposal is that, when it comes to modelling, there is a mix of more qualitative and quantitative aspects. On the qualitative side, there are certain requirements to put more governance in place. These are requirements such as the development of a risk management system and the provision of technical documentation that needs to prove compliance with the AI system. These qualitative requirements have, however, not been worked out in detail. Also, quantitative requirements, such as post-monitoring of models in production and the validation of models have not been worked out thoroughly. There seems to be a need to elaborate further on what exactly needs to be tested before putting a model into production.
Another noteworthy insight in the draft proposal is the fact that the regulation tries to come up with an exhaustive but still relatively short list of higher risk AI applications. Based on this, it can be expected that the number of high-risk applications is going to heighten at full tilt. Finally, it can be deduced from the draft proposal that a certain kind of certification process will be established similar to the consumer goods’ certification and other certifications like the ISO certification in IT security.
Chidinma: What type of organizations and applications are most heavily impacted by the draft regulation?
Jos:
The question is not as simple as it may sound. This is because this list of high-risk AI applications is still a preliminary one, and there is still going to be quite some work put into making the list exhaustive. Generally speaking, the most challenging factor is the fact that, for most sectors, there is a lack of sufficient experience in building and managing quantitative algorithms. For instance, in the banking and energy sectors, people have been using quantitative tools like models for a fairly long time. So it can be rightly believed that in these sectors it is going to be relatively straightforward to set up some additional governance. On the other hand, in sectors such as HR and mobility, organizations have not been using sophisticated algorithms extensively. Because there is less of the much-needed experience in such sectors, the implementation process of setting up certain levels of governance can be expected to be a relatively heavy exercise. They most likely have no frameworks in place and would need to set up all the basics before they can comply with the regulation.
Chidinma: What impact do you expect the regulation is going to have on how organizations can put AI solutions on the market?
Jos:
Organizations will most likely have to be organized in the way AI solutions are being built and deployed. It can be expected that organizations are going to follow a path as can be seen in the financial sector. This means that there are going to be independent teams, like the model validation team, the internal audit team, etc. Basically, a new organizational structure will need to be set up. The independent units have to verify that algorithms in their AI solutions work as expected. This is the kind of structuring that consumes so much time and energy. At Yields, we firmly believe that by putting a model risk management framework in place, organizations are going to be able to capture the value of all their analytics in a much more consistent fashion. We believe that this regulation can drive more value in the long term, but in the short term, it is going to require a truckload of additional work for most organizations in complying with the requirements of the regulation.
Chidinma: What can AI-driven companies learn from best practice model risk management in banks to comply with this regulation?
Jos:
AI-driven companies should follow the same journey as banks that have been addressing increasingly stringent regulatory expectations on model risk for the last decade. Knowing what that journey looks like makes us certain that it would be ultimately very beneficial to non-banking organizations too. To this effect, as earlier posited, each organization would need to set up a Model Risk Management framework. This framework assigns roles and responsibilities throughout the model lifecycle, defines the scope of the models, sets up the validation process, and identifies the tests that should be executed on the model before deploying it into production.
Furthermore, there are some post-market monitoring requirements as well; these are explicit in the draft proposal of the regulations and should be described in the model risk management framework. Once the framework is in place, it will become extremely natural for those organizations to start applying tools to efficiently implement the framework. This serves as a means to build a model inventory – a list of different models that fall under this regulation. With this list, the organization would be able to easily follow up on each of the models and report the models’ status to the board or the decision-makers, etc.
Additionally, the organization could also choose to create models for all the business processes related to model management. In this case, tools such as workflow engines would be necessary to actually keep track of all the processes and model-related activities.
While working with different models over time, it is pertinent to keep an audit trail at all times to be able to prove to the regulatory authorities what you have done throughout the model lifecycle. More so, when it comes to quantitative testing in heavily driven AI organizations, I would expect that they are going to have to formalize all quantitative testing as well by automating it. It can be expected that they already have certain tools related to machine learning operations in place, but these tools will have to be extended or evolved to be able to deal with the exact reporting requirements in the regulation. That is a full journey on its own, and since it is already happening in banking, AI-driven companies can easily learn from the banking sector.
Chidinma: Is there a specific threshold for AI-driven companies to work on governance, i.e., to automate validation and monitoring instead of going for manual processes?
Jos:
There is no general answer to this. Sometimes it is not just the number of models that matter, the complexity of those models is also imperative and to be considered. In some cases, there are large teams in one organization building one highly connected set of algorithms that form a single application. However, if the model itself is extremely complex with multiple teams working on it, it is still important to try to use certain tools to structure and organize the process for this, especially when it comes to all the qualitative tooling that organizations will need. An example of the needed tools is a model inventory with a task management file to keep track of all the findings. Notwithstanding that a company has a single model, it is still pivotal to keep all that information somewhere (stored in a platform) when it comes to automating all the quantitative testing. This will, however, depend a bit on how frequent things need to be done. For instance, if you think about monitoring, that is something that needs to be done continuously; for this reason, it is very sensible to always automate it. On the other hand, when it comes to quantitative testing for validation or for model documentation, assuming that you have a single model in place, you only need to produce a new version every five years. This is probably not something that you would like to automate.
The moment the cost of using a tool to automate becomes less than the cost of your manual work, then that is the moment that you should automate. Nevertheless, if you take a look at the banking sector, again as an example, you will discover that even organizations that have 5 or 10 models sometimes use a model inventory for exactly the same reason buttressed above. However, those organizations are never going to automate their quantitative testing or validation. On the other hand, once they reach about 30, 40, 50 models, then it becomes so cumbersome that they begin to automate their testing because then they can apply the same test to multiple models. This is an apt way to gain efficiency.
Chidinma: How can Yields support AI companies in addressing the requirements of the EU?
Jos:
At Yields, we are well-placed in this field, seeing as we are very much capable of helping AI-driven organizations through the entire journey. We have significant experience in creating the necessary frameworks and we have the solution in place to set up the requisite qualitative tooling like model inventory and workflow engine. Added to that, we have a reproducible data science platform – Chiron – that is actually designed to automate the model lifecycle in a reproducible and auditable manner, which is exactly what AI-driven companies need in order to comply with the requirements of the EU. In terms of AI applications, you need infrastructure to do the quantitative testing and we built our infrastructure with this in mind.